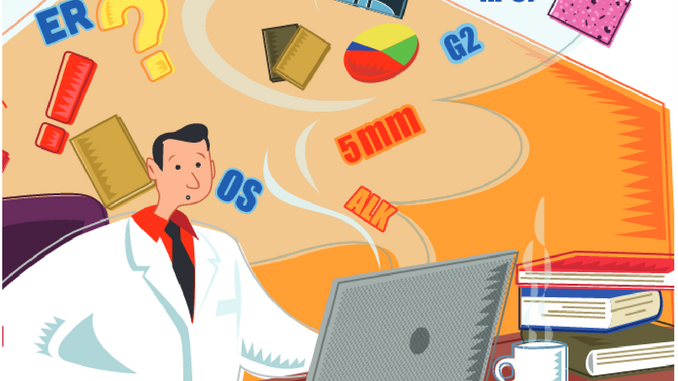
Computers are better than doctors at processing the large amounts of information involved in personalising treatments. But which decision-making tools can be relied on, and how can they best be used to help inform shared clinical decision making?
The computer will see you now.’ This was a recent headline on a British newspaper story about how a lung cancer prediction model outperformed oncologists’ predictions of survival and the chances of certain complications. This may have been a surprise to some, but for many years doctors in most branches of medicine have been using decision support tools of various types to help decide on treatments. What’s changing is the need for much more sophisticated decision tools, because diseases such as cancer now have so many more variables to consider that even experts cannot process them all without help, as the newspaper story reported.
Such is the power of these prediction tools that the developers of the lung cancer model, based at the Maastro clinic in Maastricht, Netherlands, consider that it is now unethical not to use such models for certain diseases as part of cancer care. That’s partly because the stakes can be very high in making healthcare decisions, as the correct course of action can be a matter of life or death in many situations – not least in cancer, where it is often said that there is only one chance to give the best treatment for people with disease that may progress. But decisions also affect all parts of the cancer journey, even before any diagnosis, from stopping risky behaviour such as smoking and deciding whether to attend a screening programme, to cosmetic considerations about surgery, to the particular challenges in planning care at the end of life.
But these decision support tools in cancer are still in their early stages, and while there are already many of them, they vary greatly in quality and usefulness. Given that their use is on the increase as decision making in cancer becomes more complex, it is becoming important to evaluate how they can best be integrated into everyday clinical practice.
Today, anyone can go to the Internet and find many risk and survival prediction calculators, especially for breast and prostate cancer. These tools have mushroomed in recent years as researchers have realised they can exploit increasingly rich data sets from cancer registries and other sources to calculate scores that are tailored to the characteristics of an individual. The calculators are an additional weapon in the armoury of oncologist and patient, who already have much information to weigh up, from guidelines and consensus groups, from second opinions and patient groups, and of course from the staging, imaging and genomic data from tumours. All a patient wants is the best decision about the future that suits them as an individual – and not a population group.
As Andrew Vickers, a research methodologist at Memorial Sloan-Kettering Cancer Center in New York argues, prediction is everything in cancer. “As a cancer researcher I see the disease predominately as a problem of prediction. For example, most early cancers do not cause symptoms and the reason we take out a lump is that we predict it will spread and cause problems if we don’t. And prediction is also the main problem for the spectrum of care from screening and detection to end of life decisions.”
“As a cancer researcher I see the disease predominately as a problem of prediction”
Prediction modelling started in the coronary field, says Vickers, with the Framingham Heart Study of 1948, which tested people in a town in Massachusetts and followed them for many years to see if they developed heart disease. Today there are more than ten risk calculators for cardiovascular disease, diabetes, hypertension and more on the Framingham website, and they have been the basis of much worldwide primary care decision making about say taking statins. Other heart models have been developed that give a more accurate prediction for certain populations, and have been validated in databases of millions of patients.
Cancer prediction tools have a much more recent history and, thus far, smaller datasets. As Vickers notes: “In the early 1990s it was common for a woman with breast cancer just to be handed a leaflet with a simple five-year survival risk based on only one crude variable – the stage of the tumour. It was only later in the 1990s, with the rise of the Internet and more use of computers, that it became possible to make predictions based on multiple variables as a routine part of cancer care.”
He makes the point that decisions about whether to give treatments such as adjuvant chemotherapy, based on one or two factors such as stage or lymph node involvement, “make no sense”. “Take gastric cancer and the staging about how far it has spread through the muscle wall – you can’t make the case that if it has spread you need chemotherapy and if it hasn’t you don’t – because the assumption is that no one recurs if it hasn’t spread. I’m not saying that the way we have been giving such treatments is necessarily bad, but that it makes no sense in terms of risk prediction.”
Risk prediction, he says, addresses the problem of using risk classifications with cut-off points such as stage or certain PSA levels in assessing risk of prostate cancer, where for example a high-risk classification can mask a big variation in actual risk in that group.
Philippe Lambin, head of radiation oncology at Maastricht University Medical Centre, and medical director of the Maastro Clinic, adds: “Oncology has made a lot of progress using guidelines, which are sort of recipes for treating large groups of patients, but everyone recognises we are moving to individualised medicine, which means we have to input more and more variable information and output more treatment options. As humans can only process about five variables at most, the risk is that without decision tools a patient will be subject to lottery medicine.
“The risk is that without decision tools a patientwill be subject to lottery medicine”
“Furthermore, there is now a push for shared decision making, which makes sense as with many options the preferences of the patient are also very important. Without decision support tools we just can’t say what all the likely outcomes and complications are of a series of different treatments, because doctors are just very bad at predicting the future. Making better decisions in a reproducible way independent of any one doctor is the first step towards shared decision making.”
Lambin also takes issue with the traditional international TNM staging system. “In lung cancer, which I know best, TNM doesn’t work at all for non-surgical treatments, especially when you have inoperable patients – there is no difference in survival between inoperable stage I and III after chemo-radiotherapy. TNM has been developed by surgeons for operations – it doesn’t tell you anything about outcomes after radio- or chemotherapy. And treatment decisions are not only based on survival but on complications and quality of life – even if TNM worked it is not enough.”
One of the first prediction tools that addressed more factors is the Adjuvant! program for early invasive breast cancer, developed by Peter Ravdin and colleagues in the late 1990s in the US, and since put on the Internet as the Adjuvant! Online website (which also now covers lung and colon cancers). For breast cancer, it allows oncologists to assess the risks of recurrence and dying within 10 years according to factors such as age, menopausal status, oestrogen receptor (ER) status, number of positive lymph nodes, and according to adjuvant therapy (hormonal or chemo-therapy, or both). The results are presented in colour-coded bar charts that show how far different therapy options lower the risk of death for any given set of risk factors.
Adjuvant! is not just a pioneering program, it has been widely used as a clinical tool that helps patients reach decisions on the option best for them, according to their own preferences and priorities, when it comes to balancing reduced risk against the drawbacks of a given treatment. An important randomised study showed that its use translates into women making different judgements about adjuvant therapy, because their risk is more clear; for example, those with little to gain used less therapy than the care-as-usual group. (The risk information would be even clearer, another study has found (Cancer 113:12), if Adjuvant! Online were to change the way it presents its results from bar charts to pictograms.)
Adjuvant! has been widely used to help patients reach decisions on the option best for them
Adjuvant! has also been a subject of validation work in other populations and, as with the Framingham heart model, it has been found to underestimate risk in some groups. For example, in 2011 French and Dutch researchers found that “Adjuvant! Online needs to be updated to adjust overoptimistic results in young and high-grade patients, and should consider new predictors such as Ki-67, HER2 and mitotic index” – the latter point indicating that such tools should incorporate new biological knowledge. (A nice site that has decision-making data about cancer mutations for oncologists and patients is My Cancer Genome, a “one-stop tool that matches tumor mutations to therapies,” run by Vanderbilt-Ingram Cancer Center in the US.)
Developers of other prediction tools, such as CancerMath (cancermath.net), developed at Massachusetts General Hospital, also say the algorithms they use are better than Adjuvant!, while there is also the major ongoing work on gene expression profiles (principally MammaPrint and Oncotype DX in breast cancer), which are being compared with Adjuvant! Recently, a prospective trial of Mammaprint showed good results for women avoiding chemotherapy after five years, but one expert commented that it is “hazardous” to rely only on a five-year follow up, and Vickers considers that deaths that do occur in women who forgo chemotherapy need to be carefully quantified alongside the decrease in treatment.
Another well-known set of prediction tools, for prostate cancer, was developed by Michael Katten and colleagues, and are known as the Katten nomograms – nomograms being the term for calculators that predict the probability of survival, recurrence and so on from multiple variables. But in the past few years, says Vickers, there has been an explosion in online nomograms. “It’s all too easy to set one up because all you need is a data set and statistics software – but the question is whether they can really help patients,” he says.
“It’s all too easy to set one up, but the question is whether they can really help patients”
Sloan-Kettering has a comprehensive set of cancer nomograms (at nomograms.mskcc.org), and is a leading developer of prediction tools, but as Vickers says, if you now Google ‘cancer nomogram’ you will be deluged with sites all purporting to offer calculators, and he is concerned that relying on many of them could result in more harm than good. For prostate cancer alone there are dozens of nomograms for virtually all clinical situations. In several papers, he warns that despite their “enormous promise”, most prediction models have not been validated on different datasets – which should be a fundamental principle – and that the actual clinical consequences of using models is also rarely evaluated.
For the latter point, he cites a paper that has evaluated two calculators used to help decide whether to carry out a biopsy to detect prostate cancer, which is a frequent question. It found that when an approach called decision analytics is applied, one tool would do more harm than good with most men with typical acceptance of risk. “Practical assessments of the real-world effects of prediction modeling on medical decision making and patient outcomes are all but unknown in oncology,” he writes in one paper.
Vickers is working on his own model for prostate cancer biopsy. “We have looked at more than 10,000 men so far and that’s not good enough for us to gauge how well it works. We need the same statistical rigour as when we trial drugs for good versus harm, although we may not need randomised trials in most cases.” The fact that most of the major nomogram sites also reference academic papers on which the models are based is reassuring, but the papers’ discussions inevitably also say there is much more work to be done.
So it seems that much caution is needed in interpreting the results, and this raises issues about the direct access patients and families have to many of these proliferating prediction tools, although most have strong disclaimers and some, such as Adjuvant! Online, have a registration form designed to restrict access to medics, to ensure patients review the results together with their doctor.
As Vickers says, “Before [the Internet] we were worried about oncologists making decisions without telling the patients; now we are worried about patients making decisions without telling the oncologist.”
In the case of the Maastro Predict models – currently for lung, rectal, and head and neck cancers (see predictcancer.org) – Lambin says the policy is that they should be externally validated with large datasets, and should be continually updated. To this end, Maastro and other clinics in the Meuse-Rhine region (Netherlands, Belgium and Germany) have set up the Euregional Computer Assisted Theragnostics project (EuroCAT) to develop a federated database of medical characteristics to feed into systems such as prediction programmes. While developing software products from this work is problematic as it requires costly European CE mark regulation, says Lambin, “the nomogram approach is a way of avoiding this and getting the data into the clinic.”
Although based on multidisciplinary practice, the Predict models have a focus on radiotherapy, which is Lambin’s field and the specialism at Maastro. As he explains, in recent years radiotherapy has become so complicated that old manual methods have long been set aside in favour of electronic treatment planning systems. The precision of administering radiation, he adds, is much more than can be applied for a drug in terms of how it works in the body, although progress is being made with labelled agents.
“Our rectal cancer prediction model is proving to be very useful as we can tell two weeks after the start of radio-chemotherapy treatment whether a patient will need surgery – and in this cancer, avoidance of surgery can have a massive impact on the quality of life,” says Lambin.
“Our rectal cancer prediction model tells us two weeks after the start of treatment whether a patient will need surgery”
The lung cancer story that made the news is about a study conducted by Lambin’s colleague, Cary Oberjie, who asked radiation oncologists not only to predict the chances of patients surviving for two years, based on data from more than 100 people, but also whether they would suffer from dyspnoea (shortness of breath) or dysphagia (difficulty in swallowing) after undergoing radio-chemotherapy. There are Predict tools for each of these factors. The oncologists were asked for their predictions at the time of first seeing a patient, and then again after a treatment plan had been decided. The results show that the Predict models at both points considerably outperformed the oncologists, whose own predictions were not much better than 50%, or chance.
“Our models have also been validated prospectively – they are much better than doctors and the TNM classifications,” says Lambin. He recognises though that randomised trials, comparing decision support-based treatment with guideline-based treatment, may be necessary to convince the oncology community, and that could be a next step. It is also a challenge to know how to integrate more variables, in particular genomic assays. “But I’m more a believer in non-invasive, 3D data from advanced imaging, as tumours are always heterogeneous and molecular assays are based on a random biopsy from part of a tumour. Sometimes that works, as in breast cancer, but often it doesn’t if the tumour microenvironment has to be involved.”
Further, Lambin stresses that these prediction tools should eventually be holistic in nature by integrating data on quality of life and side-effects, not least because this can improve shared decision making with patients. At present, an oncologist may need to use a range of tools to gauge treatment and complications such as acute or delayed side-effects, but he adds that at Maastro there is a prototype that brings these models into one interface.