While AI and big data are more and more used to tackle cancer, experts caution: there cannot be exceptionalism for AI in medicine. It requires rigorous studies, publication of the results in peer-reviewed journals, and clinical validation in a real-world environment, before implementation in patient care
2019 has begun with news regarding the use of artificial intelligence (AI) and big data in cancer. In the UK, the NHS has started a collaboration with Kheiron Medical to AI algorithms to try to diagnose breast cancer as competently or more than human radiologists, launching a trial on historic scans, Financial Times reports. In the US, Recursion Pharmaceuticals has announced the in-licensing of a clinical-stage drug candidate (REC-2282) for Neurofibromatosis type 2 or NF2, a rare hereditary cancer predisposition syndrome. The drug was identified using their artificial intelligence and big data platform. Many companies – including Google – are developing their own AI and data systems for a better assessment of cancer diagnosis and treatment.
Patients’ stratification
Those are not, however, the only areas within cancer management that could be benefited by the use of such technologies. Cancer stratification is also part of the list, as a necessary step for a truly personalized care. “The stratification allows further individualization of the treatment and to optimize the R&D pipeline by sub segmenting the target patients in whom the treatment behaves better”, says Ray G. Butler, CEO of Butler Scientifics.
Butler explains that his company has developed an automated, comprehensive and intelligent exploratory data analysis software – called AutoDiscovery – “that is being used by biomedical research teams in hospitals and pharmaceutical companies to discover associations of high statistical significance and great clinical relevance but that are somehow hidden in the data collected in their studies”.
One of those groups is the Translational Molecular Pathology team at Vall d’Hebrón Research Institute (Barcelona, Spain). As Butler explains, one of their goals was to assess how the immune-histo-chemical expression of the markers that are part of the signalling act as independent predictors of the risk of metastasis or variables of global survival. AutoDiscovery -he says – allowed them to find a correlation supporting their hypothesis that mTOR signalling way has a relevant role in the tumoral growth.
“One of the most valuable characteristics of AutoDiscovery is the exhaustive stratification: the ability to discover groups of patients in which exclusive associations are identified, typical of that stratum (and not others)”, Butler explains. However, the technology faces challenges that need to be considered. Among them, the identification and organization of the right data, as well as the selection of the statistical method to use.
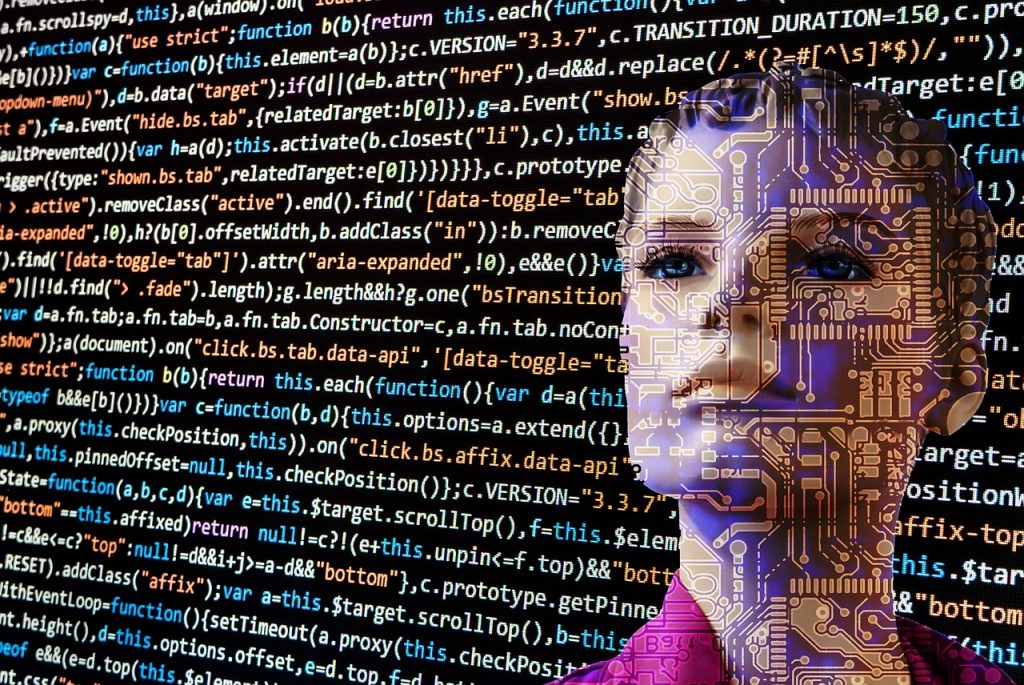
Statistical limitations
Various research groups are trying to solve those limitations. In a paper published in Scientific Reports in 2016 (Scientific Reports 2016, 6: 36493), a group from the Bioinformatics Institute at Nanyang Technological University (Singapore) show how their own model of patient classification termed Prognostic Signature Vector Matching (PSVM), combined with the classical machine learning classifiers in an ensemble voting system, “collectively provides a more precise and reproducible patient stratification”. “The use of the multi-test system approach, rather than the search for the ideal classification/prediction method, might help to address limitations of the individual classification algorithm in specific situation” they add.
Managing variety as much as volumes
There is another challenge that Butler considers more important: the adequate interpretation of the meaning of the associations. The same argues Enrico Capobianco, a researcher in Statistical Sciences at the Center for Computational Science (University of Miami, USA). “High-throughput technologies, electronic medical records, high-resolution imaging, multiplexed omics, these are examples of fields that are progressing at a fast pace. Because they all yield complex heterogeneous data types, managing such variety and volumes is a challenge. While the computation power required to analyze them is available, the main difficulty consists in interpreting the results” Capobianco writes an article published in Frontiers (Frontiers 2017, doi: 10.3389/ ct.2017.00022).
The fact that AI systems are still a black box, given their inability to explain which precise data they have used – and in which way – to get a result, doesn’t help. A way to get accountability of their decisions is needed. The industry is aware of it and there are plenty of research teams and companies trying to solve this key issue: from DARPA’s XAI project (for “eXplainable AI”) to Google’s Testing with Concept Activation Vectors (TCAV).
Also on the technical side, the availability of data could be an additional obstacle when using big data for cancer management (and for any other disease). “The key and most important element is that we must try to make all this information public and available to all cancer researchers, which will multiply the possibility of finding the essential differentiating elements with diagnostic and therapeutic potential” says Maria González Guillen, Chief Technology Officer at the oncology-focused biotech company PlusVitech, contacted by Cancer World.
Understanding cancer like never before
There are also human challenges in the use of big data for cancer management. How are physicians integrating these tools? “Using big data and machine learning (AI) methods is really allowing an understanding of cancer like never before, helping redefine the catalogue of cancers, as well as providing new opportunities for improving disease management, including therapeutic response, and even development of new therapeutic targets” assures the oncologist Ana Teresa Maia, Vice-Director of the Centre for Biomedical Research at Algarve University (Portugal).
As an example of a successful application of such technologies, Maia mentions liquid biopsy analysis: “This has been a recent improvement in patient care, as it allows a more efficient way of tracking the disease during treatment and in predicting relapse. Current efforts are very focused on single-cell approaches in which tumour cells are analysed individually, and which has the power to detect rare cells with genomic features capable of powering re-initiation of the disease even after treatment. In prior studies looking at the bulk of the tumours, these would have gone undetected and the management of the patients would be very different”.
In a paper published in Current Opinion in Systems Biology in 2017 (Current Opinion in Systems Biology 2017, 4:78-84), Maia and other authors reflect on the benefits and the challenges of using big data systems in oncology. They specifically mention the need of more sophisticated analysis tools and higher processing capacity, along with cheaper storage and faster and more efficient data transfer that must be overcome before personalised medicine finally becomes a reality. In conversation with Cancer World, Maia adds one more: the security of personal data.
“The benefits are also many, based on the very own definition of personalised medicine: the right treatment to the right patient in the correct amount at the right time”, Maia points out. “Big data and machine learning methods are helping redefine cancer. Instead of one type of breast cancer, we now can subdivide it in 11 different entities. All of them require different management because they are molecularly different. This means, as an example, that unnecessary drugs won’t be given to certain patients who are known to be likely non-responders, and just by avoiding adverse secondary effects we are already helping”, continues the oncologist.
“Some cancers might never have a cure, but certainly we are going in the direction of achieving a time in which many of them will be a chronic disease. The question is that we will keep on improving our knowledge about cancer biology and improving the technology that will allow us to look even deeper” Maia concludes.
In a paper published recently in Nature Medicine (Nature Medicine 2019, 25:44–56), the researcher and expert on individualized medicine Eric Topol, also professor, director and founder of Scripps Research Translational Institute, states that almost every type of clinician will be using AI technology (and big data as a part of it) in the future. However, or precisely because of that he cautions: “The field is certainly high on promise and relatively low on data and proof. The risk of faulty algorithms is exponentially higher than that of a single doctor–patient interaction, yet the reward for reducing errors, inefficiencies and cost is substantial. Accordingly, there cannot be exceptionalism for AI in medicine: it requires rigorous studies, publication of the results in peer-reviewed journals, and clinical validation in a real-world environment, before roll-out and implementation in patient care”.
Leave a Reply