In the golden age of computing, both the present and future of medical decision-making seem to depend on new mathematical models and systems built around artificial intelligence (AI). But how will doctors and patients feel about using (and trusting) them?
Artificial intelligence is everywhere, even in our mobile phone. And so are apps that allow doctors and nurses to take easier decisions in treating their patients, and apps that help patients to understand the pros and cons of the different treatment options. But how can this tools be used to enhance rather than replace the decision-making conversation?
“These models are good, but not good enough to be used independently”, the experts said in many articles published in the scientific literature. The application of model-based tools to aid decision-making in the field of oncology appears to be an obvious area for consideration. Nevertheless, it may prove tempting for both doctors and patients to entrust their fate in these tools. They are easy to use because they are designed for both clinicians and patients. Furthermore, they provide a rapid response, which is also assumed to be accurate and individualised, that is validated by nothing less than a mathematical model. Sounds perfect, doesn’t it? However, the reality is not such an ideal situation.
Prognostic tools
Let’s take PREDICT, an online prognosis tool, “designed to help clinicians and patients make informed decisions about treatment following breast cancer surgery”. It considers specific factors (e.g., patient age, tumour size, tumour grade, number of positive nodes etc.) to calculate survival estimates, treatment benefits for hormone therapy and chemotherapy, and mortality reductions. The only thing the user must do to obtain the predictions is entering the aforementioned parameters. Similarly, Adjuvant! has been developed to aid patients with any type of early cancer by helping them estimate the risks and benefits of getting additional therapy after surgery.
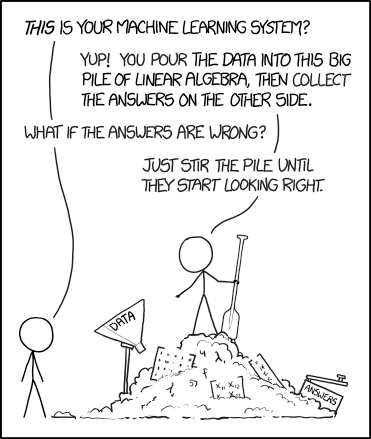
However, a recent study into both of these tools, published in the European Journal of Cancer revealed a poor calibration in the patient cohorts with the lowest and highest mortality probabilities. The authors found that both PREDICT and Adjuvant! tended to underestimate all-cause mortality in good prognosis subgroups and survival in patients under 35 years old. Contrastingly, they overestimated all-cause mortality in poor prognosis subgroups as well as breast cancer-specific mortality. Nonetheless, the researchers highlighted that, “although imprecise at the extremes, PREDICT’s estimates of 10-year all-cause mortality seem reasonably sound for breast cancer patients under the age of 50”.
Hence the first major challenge for these tools is to improve their applicability across the full spectrum of patients, according to Marjanka Schmidt, leader of the Molecular Pathology Group at the Netherlands Cancer Institute and a co-author of the study. “Such tools work well with large patient populations, but the aim is to get valid predictions for each individual person”, she says.
The second challenge, in Schmidt’s opinion, is to keep them up to date by adding important new information and factors. “There are certain changes, for instance, in breast cancer treatment modules or in the population, such as an increase in obesity, that may need to be incorporated. Obesity is a risk factor for developing breast cancer but may also affect survival. This factor is not yet included in any of these prognostic models”, she explains. In fact, Schmidt believes that one of PREDICT’s strengths is the possibility of adding this information and then validating it in an independent cohort.
Predicting recurrence with genome fingerprints
Of course, PREDICT and Adjuvant! are not the only tools of their kind, although many of the others focus solely on breast cancer. The University of Tennessee has developed a nomogram, presented in an article published in Breast Cancer Research and Treatment, to predict the probability of a patient’s breast cancer having a low or high-risk Oncotype DX® recurrence score. The calculator is available online and represents a readily available clinical surrogate for predicting a recurrence score that would otherwise have to be obtained by pathological analysis. Its purpose is to help select which patients may not require further ODX testing and act as a surrogate in patients for whom ODX testing is unaffordable or unavailable.
On the other hand, the European School of Oncology (ESO) Breast Cancer Programme coordinator Fatima Cardoso is working on a tool to complement MammaPrint (Agendia’s breast cancer genomic test that helps make treatment decisions based on the cancer’s risk of recurrence within 10 years of diagnosis). At the Champalimaud Clinical Center Breast Unit in Lisbon (Portugal) a team of researchers, led by Cardoso, are developing a model-based algorithm to increase the accuracy of MammaPrint’s results and improve its ability to predict each patient’s recurrence risk for their specific tumour.
Notwithstanding, Cardoso warns that neither this nor any other model-based, clinical decision-making instrument can be used as a fully independent tool because the decisions involved are very complex. “It is hard to translate the complexity of medicine into a mathematical language. Therefore, the model must be validated against previous patient outcomes to determine whether it provides accurate predictions that align with the real-world results. This can only be achieved with access to a good database”, she underlines.
Along the same lines, the CEO of the European Cancer Organisation (ECCO), Birgit Beger, highlights the importance of involving patients in the development of these tools (which must function transparently). For example, Beger affirms, “the increased use of Patient-Reported Outcome Measures (PROM), Patient-Reported Experience Measures (PREM) and, furthermore, the so-called patient distress scale questionnaire completed via mobile devices (smartphones, tablets, etc.) could be very important elements to ensure the patient’s voice is heard. This information, which can be collected at home and subsequently incorporated into decision-making programs at a later date. This way, physical symptoms and physical statuses reported by patients will be considered when recommending a treatment”, she adds.
Schmid agrees: “We should always take care when applying a model to individual patients because no model can be 100% accurate. I think the key issue is that a model should really be developed and validated with patient data, like PREDICT”. As with Cardoso, she also recommends that they should be used with caution, especially by patients. “In itself, it would be good if prognostic or risk tools were available online and were transparent. However, when it comes to using them to make clinical decisions, I would not recommend using them on your own, outside the official healthcare circuit”, she says.
Physicians’ and patients’ view
“If I were a breast cancer patient, then I’d want to be able to go online and look at this myself; I’d be one of those patients who would bring this information to the oncologist and say, ‘look at this tool!’”, admits Schmidt. But this is not that easy, according to Kathi Apostolidis, the vice-president of the European Cancer Patient Coalition (ECPC). “In truth –she adds- patients are not very familiar with these types of tool. What is more, they often don’t have the specific information which they request”.
Apostolidis says in Europe patients don’t trust online tools like these ones: “They need warranties that they are safe, assure their privacy, reliable, efficient, concrete and evidence based”. “Moreover, there is no regulatory framework for developers of medical apps that enables health systems, physicians and patients to trust them. Who certifies that they are safe, and reliable?”, she questions.
The ECPC vice-president states that there should be guidelines and criteria for developers of medical apps guidelines that would facilitate the adoption of these tools by health systems. “Patients and physicians recognize the advantages of these tools but in general we are conservative. Accreditation of these tools by an independent European accreditation body would facilitate their adoption and we hope that industry and developers will accept to work with criteria and guidelines. Otherwise their adoption will be delayed”, she notes.
What about physicians? How do they feel about using these tools? Eva Ciruelos, an oncologist at the 12 de Octubre University Hospital (Madrid, Spain) says she is familiar with PREDICT and others but she has never used any of them. She thinks they are useless in her daily work. “These instruments are by no means ready for everyday routine use. They do not take into account very basic factors that doctors frequently use in decision-making processes: patient preferences, previous experience, regional or local factors that can affect tolerability of or access to certain drugs, etc.”, she explains.
As an example, Ciruelos cites the case of a young patient who wishes to maintain an active working and social life, so they should not be treated according to a weekly schedule; or that of an elderly patient with little family support who lives a considerable distance from the hospital and therefore has limited access to drugs that need to be administered on a regular basis.
However, Ciruelos, who is also the Chair of SOLTI -a non-profit organisation dedicated to cooperative clinical research in breast cancer- acknowledges that tools such as PREDICT can serve as support in smaller hospitals that lack oncologists specialising in a specific type of cancer. “The final decision must always be taken by the doctor in mutual agreement with the patient”, she points out. “No digital tool can replace a doctor’s clinical judgment or expertise, nor can these instruments account for patient subjectivity or their individual conditions, which must be considered in any decision-making process. Medicine is the quintessential human profession and these platforms provide help but under no circumstances are they a replacement for doctors”, she asserts.
Meanwhile, the oncologist Marta Blanco, member of the Spanish Cancer Association (AECC), believes these systems (once they have been validated), “will offer significant assistance to healthcare professionals in terms of decision-making”. Especially when it comes to proposing treatments or monitoring patients with a risk of developing cancer. As she states, “any information based on data series derived from patient studies that are analysed according to corroborated risk models is generally helpful when it comes to supporting decisions”. This is particularly true of certain cases in which several options are available. However, Blanco affirms that these models “cannot be applied directly on an individual basis as they do not take all known risk factors (primarily clinical ones) into account when evaluating a given patient”.
Regarding the question as to whether these types of tool empower patients effectively, Blanco believes they do, “Any information transmitted to the patient helps increase their knowledge and ability to make decisions along with their healthcare team. This generates confidence in the doctor-patient relationship, which represents an additional therapeutic tool”, she claims. Similarly, Beger argues that information and knowledge are power: “Therefore, if a patient can better understand why certain treatment options are offered, that is a form of empowerment. The factors of patient involvement in design and transparency of how the system operates are both very crucial ‘ifs’ however”, she adds.
Could patient empowerment due to their use of tools such as these be a source of potential conflict with healthcare professionals? Blanco doesn’t think so, given that “the patients are generally the ones who use these systems as they have access to the data”. On the contrary, she sees them as supporting instruments that allow doctors to dedicate more time to their patients’ needs during consultations by facilitating communication.
Meanwhile Spanish general practitioner Salvador Casado doesn’t see things so clearly; he believes that it could be difficult to introduce the patient to the tool without entering into conflict with the oncologist’s opinion. “You have to consider that they could present different recommendations because oncology requires very clear explanations and the oncologist’s judgment is highly specialised”, he says. “In which case the use of these systems could complicate rather than facilitate decision making”.
Andreu Veà holds a different opinion after his brother Joaquim suffered with throat cancer for two years following diagnosis and up to his death. Veà assumed a very active role in the search for a treatment and a subsequent solution when the process was complicated by Joaquim’s allergy to a drug that could have helped cure him. He had already turned to several specialists before he found somebody who knew how to help his brother, “I would have loved it if they told me to use these tools instead of telling me to trust in a ‘good’ oncologist who cannot remember more than 80 studies”, he affirms with a certain degree of indignation.
Is computational intelligence the future?
In addition to mathematical models for medical decision-making in cancer, we can now count on a growing number of AI-based applications and systems. “It is currently a hot topic and we believe that the application of big data, machine learning and applied analytics can really help us obtain practical, real-world, clinical information”, says Cardoso. The Portuguese oncologist highlights the importance of this point because, “tt could mean practically all patients can have their own simulated clinical trial and learn what will and won’t work in their particular situation”.
From the AECC, Blanco suggests that another problem these technologies will help resolve is to deal with the growing volume of scientific knowledge, which is generated ever more quickly so it’s difficult to be up to date. A specific tool designed to help with it is IBM Watson for Oncology, an intelligent technology platform that can read a million books per second, understand natural language and learn through interaction with humans and its own environment. It can provide the optimal treatment option, according to its knowledge base, for each patient in a matter of seconds.
To arrive at this result, Watson cross-references information from each patient’s complete medical history and a specific patient questionnaire filled in by the doctor against all the literature published up to that date regarding the type of cancer they suffer. It then offers various potential treatment options for the patient, classified according to cost, effectiveness against the disease, risks associated with the treatment and the patient’s predicted quality of life.
However, before it can do this Watson needs to be trained for a period that can last several months. In fact, its development has been highly controversial. Born in collaboration with the MD Anderson Cancer Center in Texas, after four years of spiralling costs the project was abandoned by the medical center in September 2016 and is now developed in collaboration with other cancer centers in the US and abroad. The cost wasn’t the only matter of debate about IBM Watson. The outcomes of the tool were compared with the diagnosis and treatment suggestions proposed by a panel of experts. According to the first studies released, IBM Watson concordance with the experts panel is 90 percent, but experts’ panels are not considered the gold standard in medical decision-making.
The application’s critics point out its potential for bias, the lack of studies demonstrating how it affects doctors and patients, and its difficulty to learn. Blanco stresses that, “it needs to identify and homogenise useful data and information, incorporate information about the patient’s living environment, and disseminate and integrate data and information from all those involved”. Cardoso adds that the technology is “very expensive”.
IBM’s one is not the only application of its kind. Google is also dabbling in the world of AI applied to oncology. In March 2017 they published a paper in arXiv about how their deep learning technology can help pathologists detect cancer. It concluded that its method of detecting small tumours in gigapixel pathology slides “reduces the false negative rate to a quarter of a pathologist and less than half of the previous best result”. “Our method could improve accuracy and consistency of evaluating breast cancer cases, and potentially improve patient outcomes”, the authors claimed.
While these technologies present a promising future, they are both still controversial and a long way from being achieved. “Our current aim is to use them as a research tool so that in a few years’ time they may be applied in clinical practice”, says Cardoso. According to Beger, the role of technology in improving cancer care is growing and it is high on the agenda at ECCO and the next ECCO Summit. Questions remain about how to place a value on such innovations, how to leverage new technology to improve how we conduct research and the role of technology in delivering efficient cancer care.
Ethical concerns
Besides the developmental, technical, usage and practical aspects of these tools, there are still some ethical issues to be discussed. How reliable are they? Is it ethically correct to use predictive tools based on probabilities? “There is a large disparity between what these systems’ operators know about you and what you, as a patient, know about how they work”, says Itziar de Lecuona, deputy director of the Bioethics and Law Observatory (OBD) at the University of Barcelona.
“We depend on the Internet, smartphones, tablets and apps, but how reliable are these systems? How can we be sure that they interpret the data provided by each patient correctly? How are they programmed to produce reliable results?”, asks De Lecuona. She underlines the importance of the human factor in the process. “An excessive dependence on a model or algorithm is very serious, regardless of how intelligent it may be, and it is essential that the patient is aware of this”, she claims.
But De Lecuona believes another factor is even more serious; we do not know what the system does with our data, “which are probably being outsourced to third parties”. The expert in bioethics asserts that we are unaware of what this could mean in terms of our own and our family’s intimacy. “We are providing sensitive data to third parties, such as insurance companies, that says a lot about us once it has been correlated with other personal data revealed in other locations”, she explains.
While she applauds the use of these tools and the benefits they can derive, De Lecuona is critical of, in her opinion, today’s excessive enthusiasm for technology. “We need to think long and hard about the limits we wish to establish for these activities in the interest of protecting our intimacy and unveiling the opacity in which we currently live. We know very little about how to exercise our rights concerning control over our data”, she adds.
Cardoso also warns against these risks: “This is a delicate subject that must be dealt with carefully to avoid any threats to privacy. Most patients would not mind sharing their medical history and information as long as it is done anonymously and while maintaining confidentiality, but how to ensure this is one of the major challenges”, she claims.
De Lecuona’s solution is to always opt for the development of responsible innovation that meets two basic criteria. Firstly, the given systems must clearly state and explain what they do, for what purposes, to what capacity and under which guarantees. Secondly, patients must be involved in designing these models. Both requirements (transparency and user involvement in the tools’ development) are the same as those proposed by Beger and Schmidt. Schmidt considers their use ethically acceptable so long as it is in the right context, with the right information, and by a clinician who is aware of the pros and cons. “It is the best we have at this point”, she concludes.
Leave a Reply